Will Cyber Security be Replaced by AI? 🤔
AI, often perceived as that one friend who mysteriously went from novice to expert overnight, seems to have effortlessly mastered an array of skills and tasks. But is it as competent to handle cyber security operations as it appears, or is there more to the story?
It's 2023, everyone is panicking about AI taking jobs in an already competitive market, and countless big tech redundancies. Technology is evolving at such a pace that even the token over-caffeinated IT guy is struggling to keep up. AI is seemingly like that one friend who suddenly (and suspiciously) got really good at everything - at least appearing so, anyway.
But, is it time to panic? Is AI coming for your jobs? Hold your trojan horses people! Sure, AI is playing an increasingly bigger part in cyber ops. But in reality, AI is no smarter than the average toaster.
AI is actually really stupid
You don't believe me, do you? Well fair enough - ChatGPT just wrote your mate's entire uni assignment and he got an A, redesigned your company's SEO strategy and marketing campaigns so well that your boss just gave you a payrise, and it explained rocket science to your neighbour's 5 year old - so surely it's not dumb, right?
In fact, you've probably seen the countless "IQ tests" that people and organisations have put various AI models through, such as GPT-4, etc. Just Google "GPT IQ Test", there's plenty of articles/videos/blog posts/news articles boasting about AI's "above average" scores. Here's some examples:
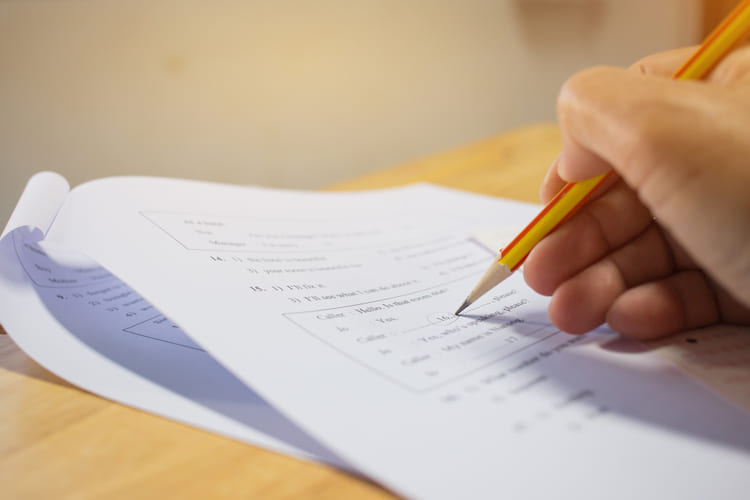

Isn't it obvious that AI is exceptionally intelligent, given all the impressive feats it accomplishes? Well, not quite. Let's break it down to 8 main problem areas:
1) Narrow Expertise
While AI, including ChatGPT, can perform exceptionally well within its designated domain of natural language processing, it lacks the broad spectrum of human intelligence. It can't, for example, help you fix a leaky tap, perform CPR, or compose a symphony, whereas we humans have the ability to adapt and excel in a wide range of activities.
2) Cost of AI Training
The training of artificial intelligence models, particularly deep learning models such as neural networks, can indeed be quite costly. This expense is attributed to various factors:
- Hardware and Infrastructure: Training deep learning models requires powerful GPUs or TPUs, and these come at a substantial cost.
- Data Collection and Annotation: Gathering high-quality, labelled data is crucial for training AI models. This process can be labour-intensive and expensive, especially for niche or specialised tasks.
- Energy Consumption: Training large AI models consumes significant amounts of electricity, contributing to the overall expense.
3) Trustworthiness of Training Data
The saying "garbage in, garbage out" (GIGO) is a common issue in AI. It is not some magical power that arrived overnight, it relies on training data. The quality of training data directly affects the performance and reliability of AI models. Issues include:
- Bias and Fairness: Biased or unrepresentative data can lead to biased AI models, perpetuating and potentially exacerbating existing societal biases.
- Labeling Errors: Incorrectly labelled data can result in erroneous model predictions.
- Data Privacy: Concerns about privacy can make it challenging to gather the necessary data while respecting individuals' rights. Ensuring the trustworthiness of training data is a critical challenge in AI, and researchers and organisations are working to address these issues through data validation, bias mitigation techniques, and ethical data collection practices.
4) New Weaknesses and Adversarial Attacks
AI systems, despite their capabilities, are not impervious to vulnerabilities. Adversarial attacks involve manipulating input data to deceive AI models into making incorrect predictions. Here's how this can manifest:
- Adversarial Examples: Attackers can subtly modify images, audio, or text to deceive AI systems into making incorrect decisions. This can have security implications for applications such as image recognition, autonomous vehicles, and more.
- AI Subversion: In some instances, attackers may aim to subvert AI systems entirely, turning them into assets for their own purposes. This could involve manipulating recommendation systems to spread misinformation or using AI-powered chatbots for malicious intents.To address these concerns, researchers are actively working on developing more robust AI models that are resistant to adversarial attacks. Additionally, organisations are implementing safeguards to protect their AI systems from being exploited.
5) Lack of Understanding
As an example model, when ChatGPT generates a human-like response, it's not a result of understanding the content in the way humans do. Instead, it's drawing upon patterns in the data it was trained on (which as covered in point 3, is not always accurate for the same reasons, such as GIGO). Sure, it can mimic intelligence, but it doesn't genuinely comprehend the concepts it's discussing.
6) No Emotional or Social Intelligence
AI lacks emotional and social intelligence. It can't empathise with your feelings or understand the nuances of social interactions. It can generate text that might sound empathetic, but it's devoid of genuine emotional awareness.
7) No Common Sense or Critical Thinking
ChatGPT often struggles with common sense reasoning and critical thinking. It can provide plausible-sounding answers even when they're incorrect or nonsensical. Human intelligence relies on a deep understanding of context and the ability to critically evaluate information.
8) Limited Creativity
AI can assist in creative tasks, but it doesn't truly innovate or have original ideas. Any creative output from AI is a result of blending existing patterns or data, not true inspiration or artistic creativity.
TL;DR
AI can excel in specific areas but lack the comprehensive, adaptable, and multifaceted intelligence that defines human cognition. In fact, chances are, the AI model was trained on the IQ test data, or at least a very similar version. You would look intelligent if you had and/or could predict all the exam answers too. Most of the IQ tests are predictable, or have already been analysed millions of times by the model. In fact, the data they were trained on might not even be reflective of real-world conditions.
Relying soley on AI for Cyber Security is a terrible idea
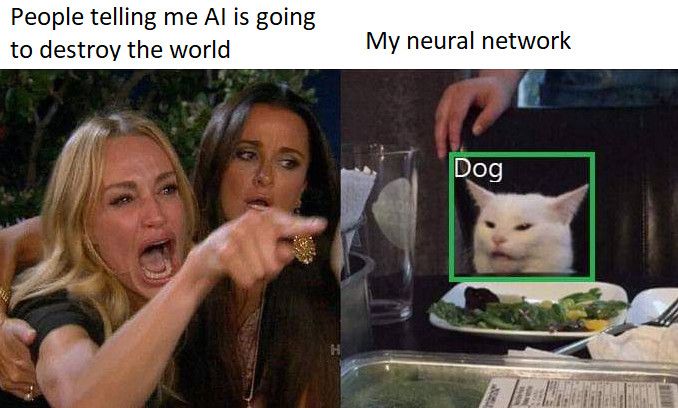
Okay, I just want to preface this section by saying machine learning (or AI) is actually a very helpful tool - but let's not pretend that it has any intelligence (hence Artificial Intelligence), self-awarness, or conciousness - this is why relying soley on AI for cyber security is a terrible idea, and will never work. Though, there are some essential elements of cyber security which AI is good at in this context, given its fast ability to analyse and find patterns in data:
1) Threat Detection and Analysis
AI excels at sifting through enormous volumes of data to identify patterns and anomalies. It can continuously monitor network traffic, log files, and system behaviour to detect unusual activities that may indicate a cyber threat. This speed and scalability make it invaluable for real-time threat detection. Check out this article from Forbes:

2) Behavioral Analysis
AI can analyse user and entity behavior to establish a baseline of what is normal in a network or system. When it detects deviations from this baseline, it can trigger alerts, helping cybersecurity teams investigate potential threats more efficiently. This article from Crowdstrike (2023) explains it really well:
3) Predictive Analytics
Machine learning models can predict potential vulnerabilities or attacks by analysing historical data. They can provide early warnings and enable proactive mitigation measures. Muhammad Hassan (2023) explores this further:

4) Malware Detection
AI-powered anti-malware systems can quickly identify and quarantine known malware strains and even identify new, previously unseen threats based on behavioral patterns. Isla Sibanda (2023) recently explored advanced malware detection using AI-powered tools:
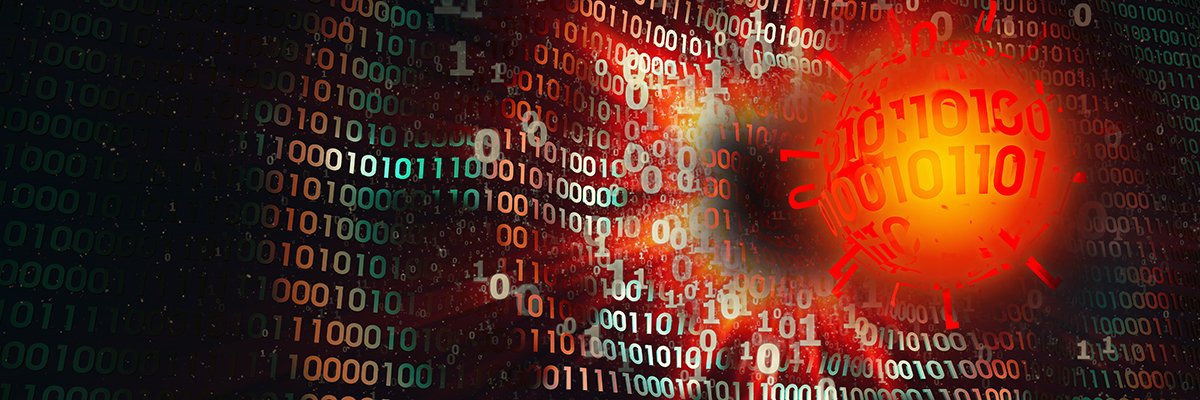
5) Phishing Detection
AI can analyse email content and sender behavior to identify phishing attempts, protecting users from clicking on malicious links or downloading infected attachments. Phishing usually effects our most vulnerable users as well as businesses. According to IBM (2023), the global average cost of a data breach in 2023 was $4.45 million USD, with around ~36% of all data breaches last year involving phishing (Cveticanin, 2022).
A really amazing preliminary paper on detecting phishing attacks using machine learning from the University of North Carolina found to be highly accurate;
"high accuracy is reported by Gaussian Radial basis function kernel. For LR, the high accuracy is given by a regularization parameter corresponding to 0.4. For ANN, high accuracy is achieved with two hidden layers" (Salahdine, et al., 2023)
6) Incident Response
AI can assist in automating incident response processes, helping organisations react more swiftly to security incidents, isolate affected systems, and minimise damage. Adam Zoller, CISO for Providence, said in a recent conference:
“as a human running a human team, we are not equipped to operate at the velocity of what our attackers are going to bring to us in the next two to three years.” (VentureBeat, 2023)

7) User and Access Management
AI can help in authentication and access control, ensuring that only authorised personnel gain access to sensitive data and systems. It can detect unusual login patterns and take action to protect against unauthorised access. A recent article by Forbes (2023) covers some pros and limitations with this:

8) Security Analytics
AI-driven analytics tools can provide security teams with actionable insights by processing and correlating vast amounts of data from various sources, aiding in threat hunting and decision-making. Synk has an amazing article on security analytics in combination with AI:
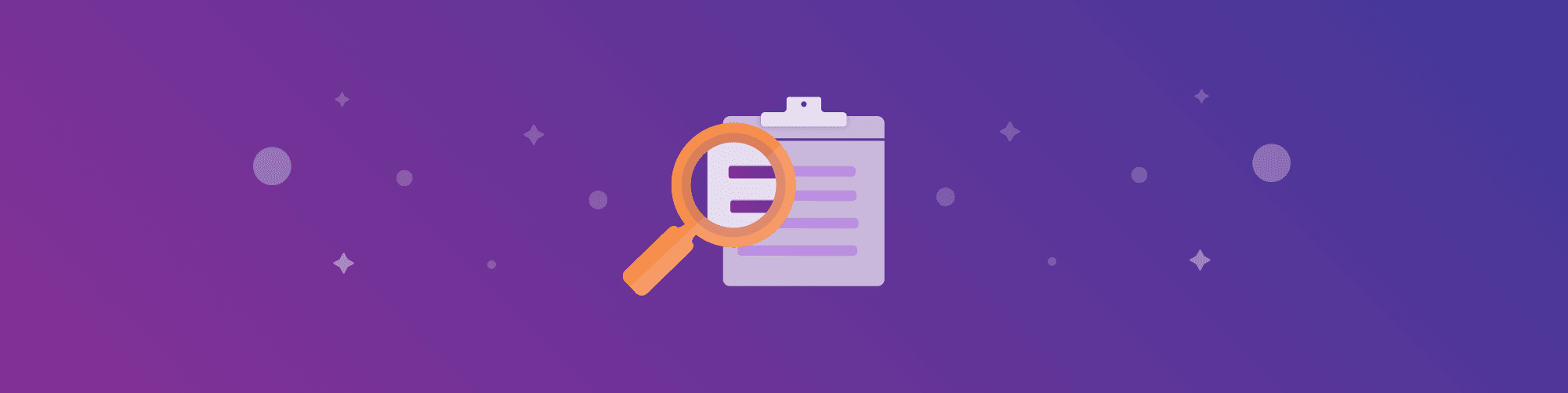
9) Anomaly Detection
AI can flag unusual system or user behavior, allowing security teams to investigate potential security incidents promptly. Microsoft has already implemented this tool in its Azure platform:
10) Automated Patch Management
AI can assist in identifying and prioritising software vulnerabilities, making it easier for organisations to patch critical systems promptly. IT Pro released an article last week on how AI is changing patch management, including the risks of AI involving patch management:
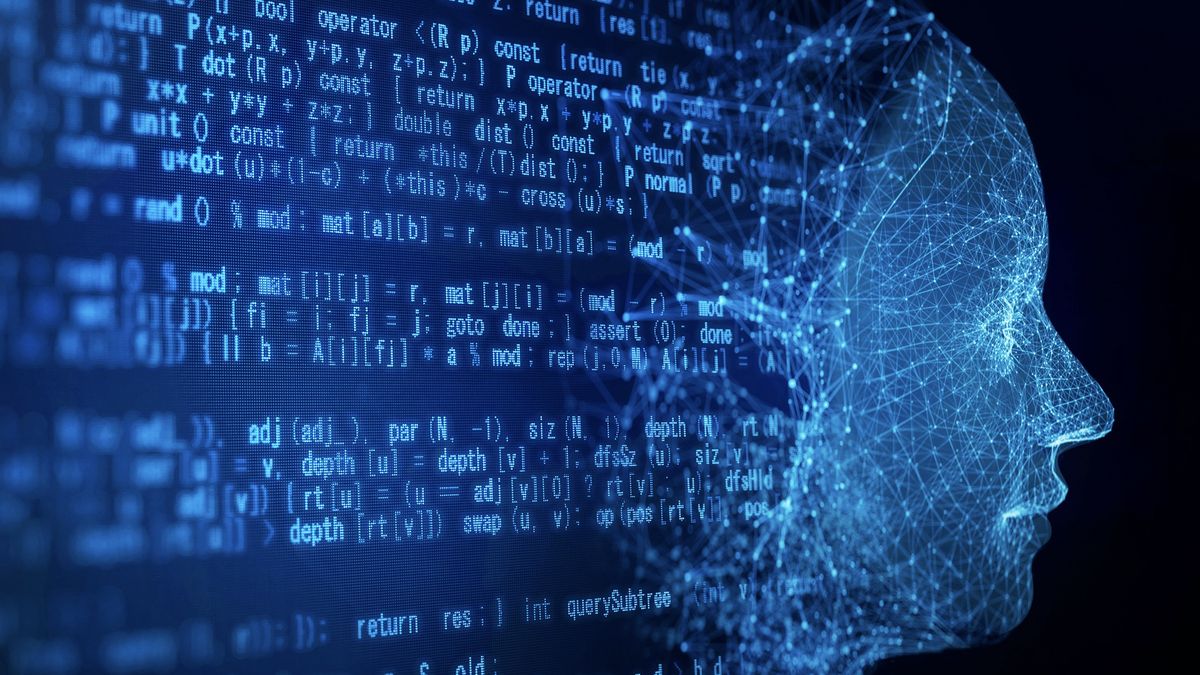
Why your Cyber Sec job is still safe
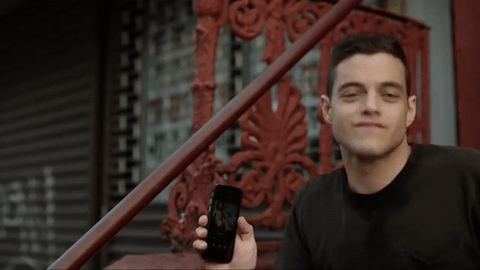
To the untrained eye, it's tempting to think that AI is on the verge of taking over the world, one job at a time - especially given the media's perception and mass panic/accptance - but, let's not pull the cord just yet. As we've seen, AI might be the star of the show in certain areas, specifically data analysis, but it's not exactly winning any awards for intelligence.
Sure, it can mimic human-like responses, generate creative ideas, and even help us in various aspects of cybersecurity. But beneath that shiny facade, AI lacks the depth and breadth of human intelligence. More specifically:
Contextual Understanding
Humans can understand the broader context of a cybersecurity incident, which AI may lack. They can discern between a false positive and a genuine threat, taking into account the business impact.
Adaptability
Cyber threats are continually evolving, and human expertise is crucial for adapting defenses and strategies accordingly.
Ethical Considerations
AI may inadvertently introduce biases or make decisions with unintended consequences. Humans (i.e. you and I) must ensure the ethical use of AI in cyber security, as with any data AI models are allowed to touch.
That being said...
AI tools are valuble, but it's not about to replace the human touch anytime soon. We're still the ones with the big-picture understanding, adaptability, and ethical compass needed to navigate the ever-evolving landscape of cybersecurity.
Embrace the technology, use it to your advantage, but remember that when it comes to the most critical aspects of cybersecurity, human expertise and oversight will always be indispensable.
Of course, there's always a balance to be had between 'keeping up' and applying AI too quickly. Involving any of these tools requires full knowledge of a specific system structure, its complexity, and pros vs cons when involving automation. It requires a true industry professional to make the right decision.
As always – stay safe out there.
Thanks to Andy Farnell, Stetson Blake & Darrell Bos for edits/suggestions.